More and more applications are leveraging the power of machine learning and neural networks for object recognition. However, how efficient artificial intelligence is at such tasks depends, among other things, on the quality of the training data. With the right tools, suitable data sets can be generated via tablet and smartphone.
Is the road clear or is there an obstacle crossing the travel path? Do the corn plants need more water? Where is the next screw for the robot to grab and insert? Automated analysis of image data has become a domain of artificial intelligence (AI). The range of applications extends from visualization solutions in industry through recognition tasks in medicine, agriculture and logistics to autonomous driving.
Neural networks, i.e. networks that are based on the structure of the human brain, are particularly suitable for object recognition. But the network architecture is only part of the secret to success. These networks first have to be trained for the specific tasks using machine learning. This requires suitable data sets made up of images and informative descriptions. The quality of this training data is one of the key factors in determining the subsequent recognition performance in real operation.
Traditional Data Acquisition
For numerous everyday objects - people, animals, cars and many more - high-quality training datasets already exist and are freely available online. In logistics and industry, however, data sets are always needed for new objects. Until now, generating this kind of initial training data has been extremely complex. The underlying procedure is called data annotation.
This involves specifying certain conditions or representations that a data object must meet. These conditions are determined by the nature of the neural network and the training process. The annotations for the corresponding images are then generated. To do this, it is necessary to mark the desired object in the image material by means of bounding boxes and to supplement these images with further data, in particular object designation or object class, but also further data for describing the object or its context, such as size or position.
There are already a variety of solutions and approaches to data annotation. In particular, the following five procedures are the most common in practice:
- Single data generation: Manual annotation of individual images from a video, known as frames;
- Crowdsourcing: Projects to merge data sets using single data generation across multiple sources;
- Partial automation procedures: Data generation using propagation, copying annotations for static objects, or linear interpolation of moving objects from single frames of videos. Tools such as object trackers or pre-trained models provide more intelligent support - provided that suitable data and models for the objects in question already exist;
- Data augmentation: Artificial augmentation of existing datasets, for example by rotating, mirroring, distorting, or blurring annotated images;
- Synthetic data: Automated generation of random training data using modeled objects.
In part, these procedures can only build on existing data. Others require too much time to generate sufficiently large data sets. Especially for areas and objects that need to be developed from scratch, such as new types of products in the logistics center, other ways of data annotation that allow the solution to be put into operation in a timely manner must therefore be found.
Ways to Mobile Data Annotation
Mobile devices such as smartphones or tablets have at least one but sometimes several high resolution cameras, as well as constantly improving computing power. This makes them ideal for data annotation: they can take photos and videos of the objects and execute tools to support annotation, such as object trackers and segmentation algorithms. Thus, users can annotate the object shown in the camera image of the mobile device with a bounding box. The segmentation algorithm further adjusts the bounding box based on unique features. The object tracker can be used to track the object across frames, i.e. from image to image.
The object can therefore be photographed from various angles and distances, while the bounding box is defined just once, when recording is started or optimized by occasional re-segmentation and adjustment of the bounding box. Once an object has been photographed from every perspective, the process is repeated for the next object until a sufficiently large training dataset has been collected. Before uploading the data, for example to cloud storage, individual off-center annotations or blurred images can already be sorted out. In this way, the quality of the training data is improved at an early stage. Likewise, the training data can be used to pre-train a neural network and the result can be used on the mobile device. Firstly, to test the recognition quality, and secondly, for automated data annotation, which simplifies the further collection of training data.
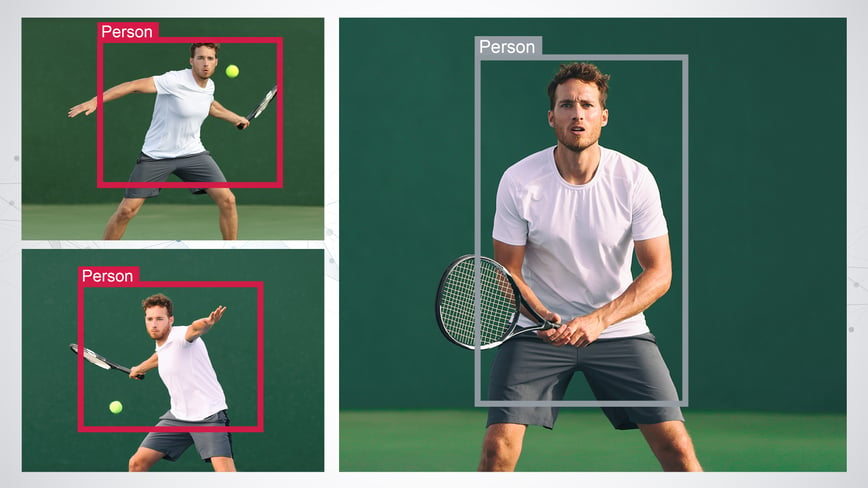
Customized Technology
The technical specifications of the mobile device must be considered. As a general rule, a tablet is more suitable than a smartphone, thanks to the larger display and better performance. Similarly, greater success can be achieved by using different object trackers, segmentation algorithms, and machine learning models. This makes it possible to respond to changing situations - from lighting to object size and type to weather - while the system is in operation. This procedure promises greater efficiency and higher quality annotations.
There are also applications in which the integrated camera cannot be used, such as in medical imaging procedures. However, the basic concept can be applied here as well, with live data from ultrasound, MRI or PET-CT being highlighted by medical staff during routine examinations, in order to distinguish between healthy and abnormal tissue. In this way, the necessary training data can be generated for AI models that later assist in the detection of diseases.
Broad AI Skills
EDAG Engineering has gained extensive know-how in the field of artificial intelligence in recent years. The focus here is not only on practical applications. The AI experts at EDAG also advance the development and optimization of concepts and processes from different disciplines. One such example is the efficient generation of training data for neural networks using mobile devices. If you have any further questions on this topic, please contact Maximilian Happel, Development Engineer in the Concept Development department at EDAG Engineering. Or download the detailed whitepaper here: "How to Quickly and Efficiently Generate Training Data for Neural Networks".